The rapid deployment of artificial intelligence (AI) today across new domains of society, including key services, state administration, and social frameworks, invites us to consider AI as an emerging form of comprehensive infrastructure and a system of political governance.
Such algorithmic architectures have previously been discussed as engendering a new form of political society. In 2006, the sociologist A. Aneesh, coined the term algocracy to describe how the covert design of algorithms tacitly shapes behaviors, bypasses traditional governance, and asserts authority without public awareness or debate.[1] Aneesh was writing in a late-20th/early 21st century context of labor and migration between the US and India in which algorithms, although not necessarily AI algorithms, were redefining workers’ conditions, employment rights, and immigration status. Today, his ideas take on advanced meaning through the prospect of larger scale algorithmic infrastructures and governance.
Far from discrete algorithms developed by individual developers, AI frameworks need to be understood as larger infrastructure projects that are fueled by significant investment into dataset creation, including the labor of labeling, as well as thousands of hours of model training. Beyond a materialist analysis of AI’s infrastructure, we can consider dataset creation and model training as defining a specific ontology, registering and rendering visible an inventory of phenomena within a specific algorithmic system. Such ontologies are explicitly shaped at the moment of labeling data and should be understood as the result of a soft infrastructure established through the gaze of the labeling agent.
We live in a pertinent moment in which CEOs of the largest US technology corporations are urgently, and unusually, requesting regulation on the further development of their AI products. Simultaneously, the US government has requested the public to suggest ideas for policymakers on how to develop AI accountability measures and fast-track the writing of an AI Bill of Rights. AI models occupy unstable ground; as uninterpretable generative systems they hold an ethical contention at their core, they raise questions about the role of human agency in the future of human organization and governance, and they struggle to negotiate social justice as a more complex socio-technical problem.
Within this context, my research attempts to conceptualize what I term Unmodelled. It is a critical computational strategy that foregrounds the values that are missing from computational models. It renders visible the absence of specific data features, like transversal ghosts, that haunt the data structure. This strategy seeks to expose arbitrary ontologies embedded in training datasets and reveal alternative ontologies that exist in the blindspot of established practices. It is an experiment that emerges from counterdata practices, which give materiality to the absence of datasets, and then extends this to think about modeling. Unmodelled visualizes considerations missing from a model, and it is a design strategy to analyze and reimagine how human values could be encoded into algorithms.
Unmodelled is used as a methodology to contest established worldviews and open a process of public deliberation. As a project, it seeks to re-root critical art practices as contributors to such soft infrastructure, arguing that a process of interpretability and contestation of a fixed ontology cannot circumvent humanistic thinking.
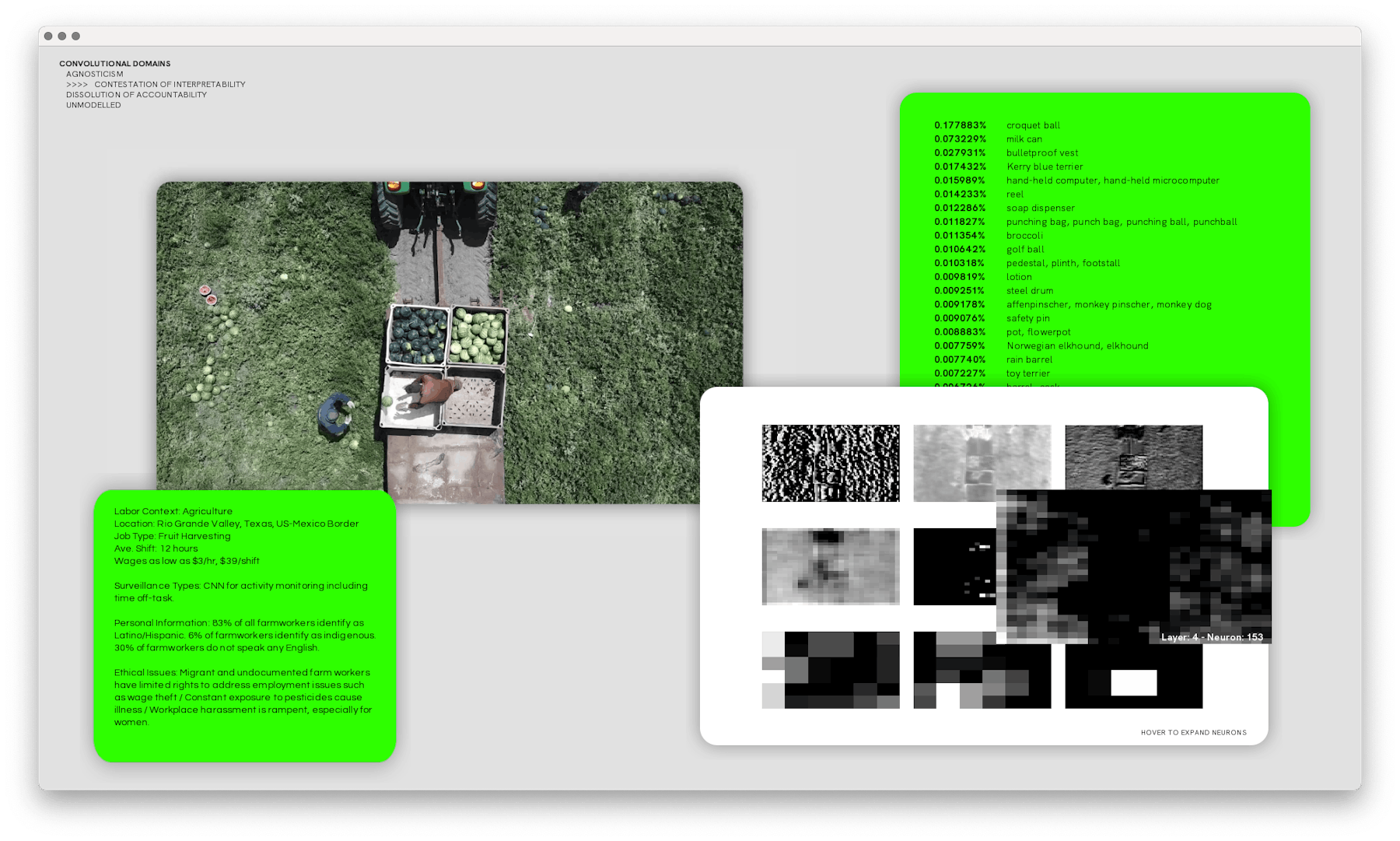
[Fig. 1] Convolutional Labor Domains, an experiment in reflexive software development.
Unmodelled is a software interface and the third chapter of a larger creative research project titled Convolutional Labor Domains. It is also a component of a broader methodology in reflexive software development. Where traditional software development prioritizes operational tooling and a seamless user experience, reflexive software offers the user an experience of how our algorithmic infrastructures might be understood as ideological and thus contestable. The approach is what James J. Brown, author of Ethical Programs, calls computational rhetoric, a strategy of building arguments in and through software design, rather than about software.[2] Figure 1 displays an interface that both visualizes the inner workings of an AI model and situates it in a context of contestation. A video feed displays workers in the agricultural industry, and a sample of nine nodes from the network analyzes the image and predicts a list of possible objects along with a percentage of certainty. Alongside this process, a series of facts about the agricultural industry, worker conditions, environmental situation, and socio-political situation is revealed as an alternative analytical orientation.
The broader research frames a near-future scenario in which existing prototypes for autonomous worker management systems are applied in workplaces to monitor worker productivity, under the guise of worker health and safety. Human activity recognition (HAR) algorithms are an emerging suite of machine learning models gaining traction. They surveil the body’s movements in intricate detail to discretize behaviors, predict worker actions, and evaluate their efficiency. HAR algorithms form part of a wider discourse about the use of AI in the workplace and its impact on worker rights and labor relations.
Emerging from the field of critical data studies, Yanni Loukissas advocates for counterdata practices that “challenge the dominant uses of data to secure cultural hegemony, reinforce state power, or simply increase profit.”[3] Loukissas asks us to create datasets that provoke, agonize, and counter traditional narratives about data collection and its uses. As a strategy, counterdata has various affordances, including the way it can encode different perspectives into a problem, counteract dominant historical representations, and contest normative, top-down, and institutional approaches to data collection. It also supports the reimagining of alternative data futures.
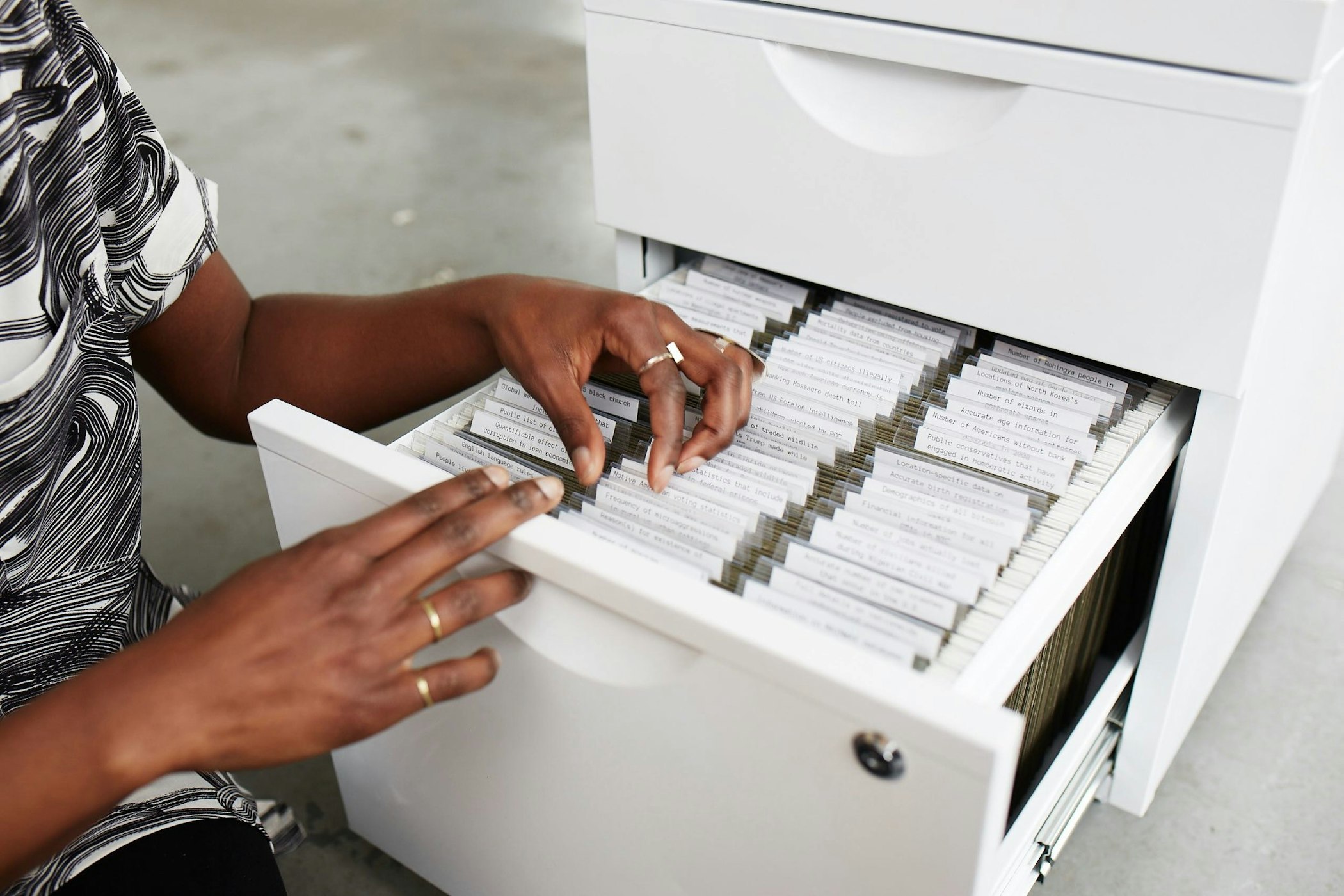
[Fig. 2] An installation view of The Library of Missing Datasets by Mimi Ọnụọha.
The art research project The Library of Missing Datasets by Mimi Ọnụọha [Fig. 2], takes the form of a physical installation, comprising a traditional office filing cabinet that contains rows of manila file folders each labeled with the title of a dataset. Example labels include: ‘Total number of local and state police departments using stingray phone trackers (IMSI-catchers)’, ‘How much Spotify pays each of its artists per play of song’, ‘Master database that details if/which Americans are registered to vote in multiple states’. However, upon closer inspection, all of the folders are empty, and the labels actually describe datasets that do not exist. According to Onuoha, “[t]hat which we ignore reveals more than what we give our attention to. It’s in these things that we find cultural and colloquial hints of what is deemed important. Spots that we've left blank reveal our hidden social biases and indifferences.”[4] In a data-saturated world, missing datasets signify exclusion and normativity, and they point to the power asymmetries that mask the advantage to some groups that certain data remains uncollected.
Can we map counterdata approaches into countermodels? Models are important devices to contend with complex issues: the effects of climate change, the impact of a policy decision, predicting disease transmission during a pandemic, and more recently, a key function in machine learning software. Models are also always wrong; they are incomplete and come at a cost, according to Scott Page, author of The Model Thinker and Professor of Complexity, Social Science, and Management at the University of Michigan. Page proposes “many-model thinking” as an ensemble approach to modeling, one that could overcome a single model’s blindspots and limitations.[5] Whilst the Unmodelled project could be an exploration of a many-model thinking approach, it also recognizes that not all phenomena can be reduced to the mathematical. Unmodelled leaves space for the incommensurable.
I conceived a set of unmodelled parameters, or missing datasets, across disparate places: existing metrics in subjective wellbeing research; existing research into the context of workers, labor rights, and relations; and investigations that forecast the implications of AI in the workplace, such as Poverty Lawgorithms by Michele Gilman, which looks at the new frontier of workers’ rights and digital wage theft and how workplace algorithms are programmed to create information asymmetries by favoring the employer and being impervious to the employee.[6] Additionally, I modified data parameters to create a connection between subjective wellbeing in a labor context and critical AI studies, and I speculated on new datasets that do not exist but would radically shift how we perceive human values to be modeled by algorithms. By partially drawing on subjective wellbeing research as a counterdata strategy, the Unmodelled becomes a speculative dataset that agonizes the future of work and the promise of AI. Additionally, the subjective nature of wellbeing data conceptually counters the presumed hierarchical information structure in workplaces from top-down to bottom-up.
Figures 3 through 6 display software interfaces that speculate on unmodelled ontologies for four different labor domains, including Agriculture, Textiles, Warehousing, and Construction. These interfaces visualize counterdata parameters as a speculative ethics to question whether different value systems can be learned by different machine learning model designs. Taking the form of outliers—non-equal and non-correlated features—they are an experiment in what Loukissas calls interfaces that cause friction in order to recontextualize data’s uses and abuses. By visualizing the gap between meaning and function in a machine learning model, they bring deliberation to many-model thinking. Unmodelling points the way toward a practice of counteralgorithms, or algorithms designed to challenge, provoke, and counter normative assumptions built into software infrastructures.
Unmodelled opens up resistance to simplistic models with messier, contextually grounded lived experiences to address more complex, nuanced, and socially sensitive ethical considerations around AI in the workplace. The Unmodelled point to the advantages of their absence to those in power, revealing whose priorities are being modeled and whose are not.
Such counterdata parameters are not proposed as a corrective solution to a productivity algorithm. The project does not advocate solving the problems of labor rights and relations with a better algorithm. Unmodelled parameters are designed to haunt the vision of labor management, productivity, and convergence—as we transition to Industry 4.0—with concepts of wellbeing and an ethics of subjective life satisfaction. What could the future of work look like through the lens of subjective wellbeing versus AI productivity monitoring? Is a human-centered artificial intelligence possible that optimizes for subjective wellbeing, or is such a proposition a distraction from the harms that widespread AI in the workplace could cause? How can we conceptualize machine learning beyond only accuracy, optimization, and homophily?